One of the fundamental goals of materials science is to learn structure-property relationships and to discover novel materials with desired properties from them. Previous efforts have mainly relied on chemical intuition and empirical rules taken from the prior knowledge. However, in the conventional approach, the results vary person to person and can also be slow. Such inverse design of new materials with the pre-defined desired properties thus has been the major focus in recent years, but demonstrating such a possibility for inorganic solid-state materials has been challenging due partly to the invertibility of representation used for machine learning models.
The collaboration of a KAIST research team led by Professor Yousung Jung and University of Toronto research team led by Professor Alan-Aspuru Guzik proposed and reported the first autoencoder-based generative framework (iMatGen: Image-based Materials Generator) for designing inorganic materials in Matter on November 6, 2019. The model was possible by introducing an invertible 3D image-based representation of inorganic crystal structures.
The ability of autoencoders to generate experimental materials was first demonstrated with vanadium oxides via rediscovery of experimentally known structures when the model was trained without them. In addition, the research team successfully generated several completely new computationally meta-stable vanadium oxide materials that may be synthesizable by sampling approximately 20,000 hypothetical materials from the learned continuous latent space. Comparison with genetic algorithms suggests computational efficiency of generative models that can explore chemical compositional space effectively by learning the distributions of known materials for crystal structure prediction.
Prof. Jung said "generative frameworks have tremendous potential in the field of materials discovery since they allow data-driven navigation of the hidden portion of the chemical space, and the proposed autoencoder is only a beginning yet important first step towards accelerated materials discovery using generative approaches".
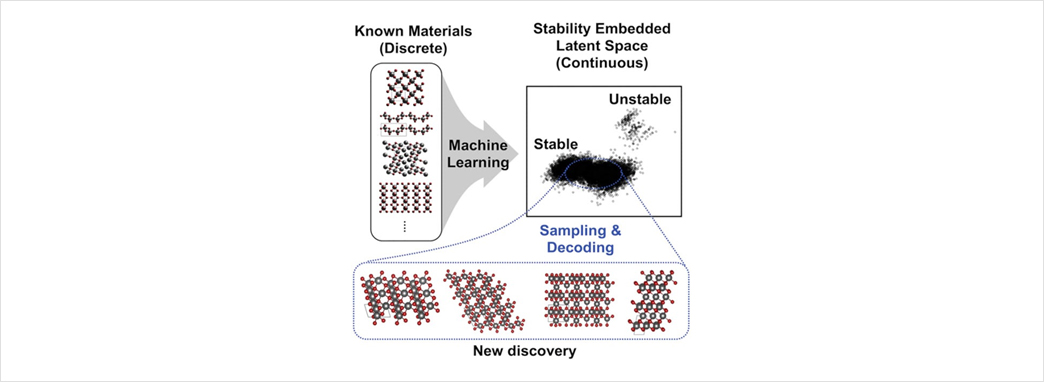